How to structure UX research
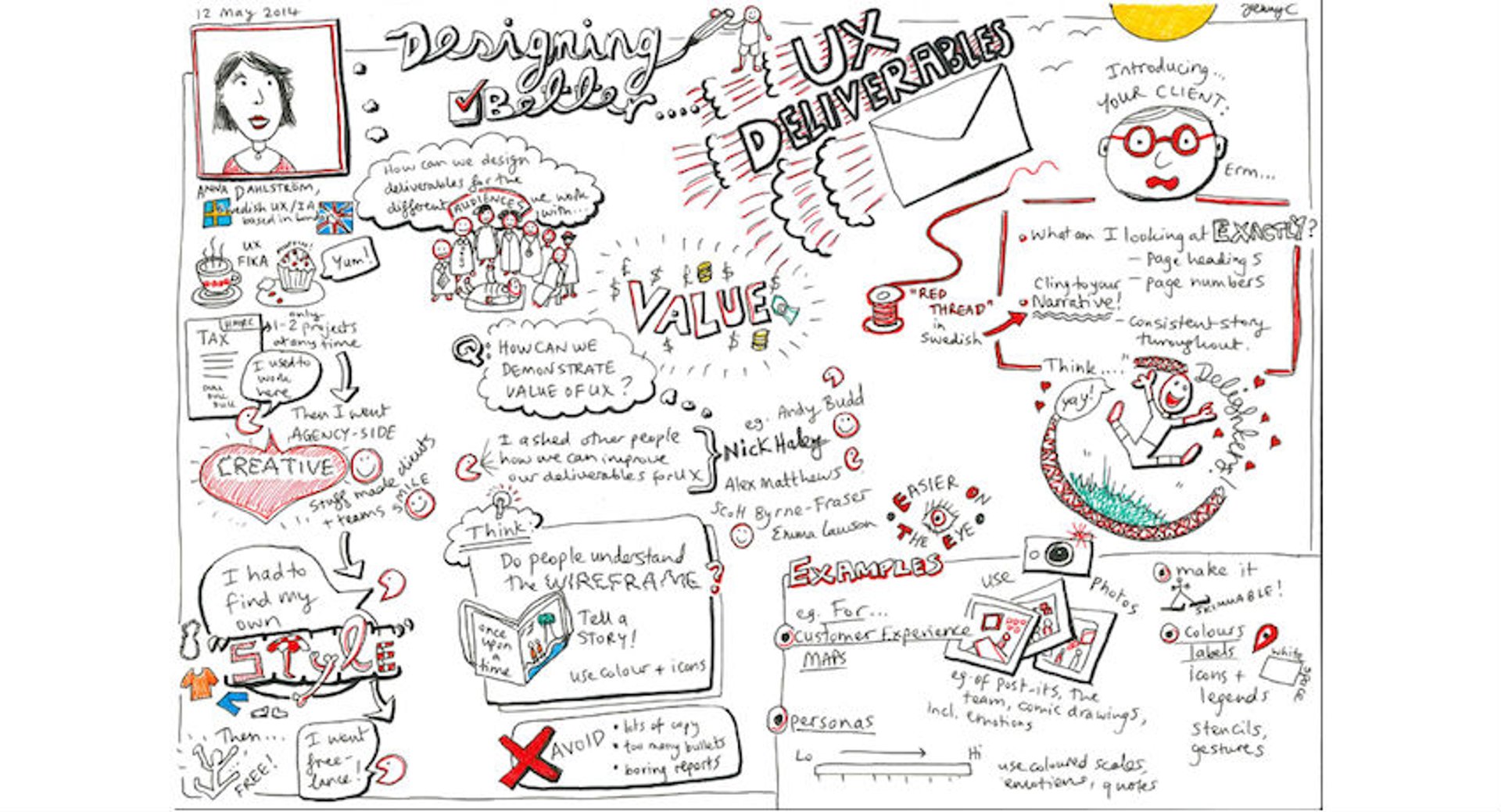
Published 2023-07-20
Summary - Looking for ways to improve your product? Good, science-based UX research will help pinpoint problems and direct you to solutions.
It is critically important to track the experience users have with your product. If you don’t know where your users struggle, and why, you won’t know where things can be improved.
That’s where UX (user experience) research comes in. It offers a systematic way to extract insights from empirical evidence through your design and development process. And the best part is that, to paraphrase Steve Krug, it’s really not rocket science.
This post is intended to show someone new to the concept of UX research how to bring more data-driven decision-making to your organization though UX research. We’re using one of our own experiences as a case study to provide an example of what can be done.
The basic concept of UX research
While the process for conducting UX research is fairly fluid in practice, it can generally be broken down into a cycle alternating between:
- Generating a hypothesis
- Testing the hypothesis
When investigating an issue, you’ll go through cycles of hypothesis generation and hypothesis testing. At each stage in the cycle the focus will narrow until you end up with something very specific.
Keep in mind that qualitative methods tend to be better for hypothesis generation, while quantitative methods can be better for hypothesis testing.
The case study
The issue we were dealing with was conversion. We were concerned that our trial customers were converting to customers at a rate that was lower than we expected.
Our challenge was to find out why that was happening, so that we could then address the issue and correct any problems.
Starting point: Generating an initial hypothesis
We needed a starting point for our research, so we needed to generate an initial hypothesis about what was wrong.
To do that, we started by doing some semi-structured interviews, talking to people who participated in trials but who did not convert. By "semi-structured" I mean that we had a framework of questions, but let the participants guide the conversation.
Even in just a dozen or so conversations, we found a clear theme. Users liked the power and flexibility of our tool, but they found the learning curve very steep. They were also scared of the time they needed to invest to get set up.
The issues largely boiled down to their experience in the Editor portion of our tool.
We had our hypothesis: The Editor (an important part of unlocking Klipfolio’s full potential) was too hard to use.
To provide background and context, we also talked to successful trial participants who had recently converted into paying customers. We heard something similar from them. These users often spent a lot of time getting up to speed, even doing extended trials and spending hours outside of their regular work time learning the tool.
Tip: Interviews have their weaknesses, but they’re a great asset when used in the right places in your research mix. Here's some advice from the Nielsen Norman Group on how to do it right.
Validating the hypothesis
To validate our hypothesis, we sent out a survey to a broad set of customers. We wanted to know if other people felt our Editor tool was hard to master.
They did. Overwhelmingly. In fact, the answers to the survey were very one-sided.
As a result, we were able to validate our original hypothesis that the difficulties trial users were experiencing with Editor was a main reason why they did not convert into customers.
We ended up making a similar survey a standard part of our cancellation process and we track it on a dashboard for everyone to see.
Tip: Be careful not to ask leading questions! If you ask your users, “Does it bother you when the application is slow?” the answer will inevitably be “yes.” But you will get a more useful answer if you ask them which issue among several was the biggest reason for them deciding not to convert.
SurveyMonkey has some introductory advice for survey design here.
Zeroing in on the problem
At this point, we knew that the Editor tool was a problem, but we did not know exactly what about it we could fix. To find out, we conducted some usability testing.
By observing five users trying the most basic task in our tool—to make a bar chart of some values—we were quickly able to see where the issues were and develop a hypothesis for how we could solve the problems.
We supplemented this with our own heuristic evaluations and expert walkthroughs, creating a map of the low points in the user experience. We used that map to come up with a roadmap of fixes that would transform the overall experience over a period of time.
Tip: A great introductory book for learning usability testing is Steve Krug’s Rocket Surgery Made Easy.
Testing your solutions
Just because you think you have found a solution doesn’t mean the problem is solved. We needed to test our solution with more usability testing.
When first observing users we are often surprised because we see behaviour that contradicts our expectations and generates new ideas. With time, however, patterns emerge. After making changes to the user interface and running the same usability test again, it is possible to observe how behaviour and outcomes change (or don’t).
Tip: You only need three to five participants to uncover 80% of the critical issues within the tasks you test. This means it’s easy to move very quickly and iterate often. But be sure to be very clear about what kinds of users you want to test. With small sample sizes, not testing the right users can make a big difference.
Monitor changes in behaviour as you roll out solutions
Usability testing our new designs allowed us to derisk moving on to the next stage—getting changes out to customers.
The changes we planned to the Editor tool were extensive, but we made a point of rolling them out in bits and pieces so that we could pinpoint exactly what change was having the greatest effect.
A good tool in this regard is A/B testing. By randomly assigning users to groups that experience changes (or don’t), or by creating a number of groups with different changes, we were able to isolate the effect of each change by comparing the groups.
We observed a significant increase in a subjective satisfaction questionnaire administered in Editor.
Tip: There are many ways to gather ongoing metrics on your users and to conduct A/B testing. For tracking we use Mixpanel and Google Tag Manager. For this particular A/B test, we implemented our own random assignment and used Mixpanel to track the data, although we make extensive use of Optimizely for smaller changes.
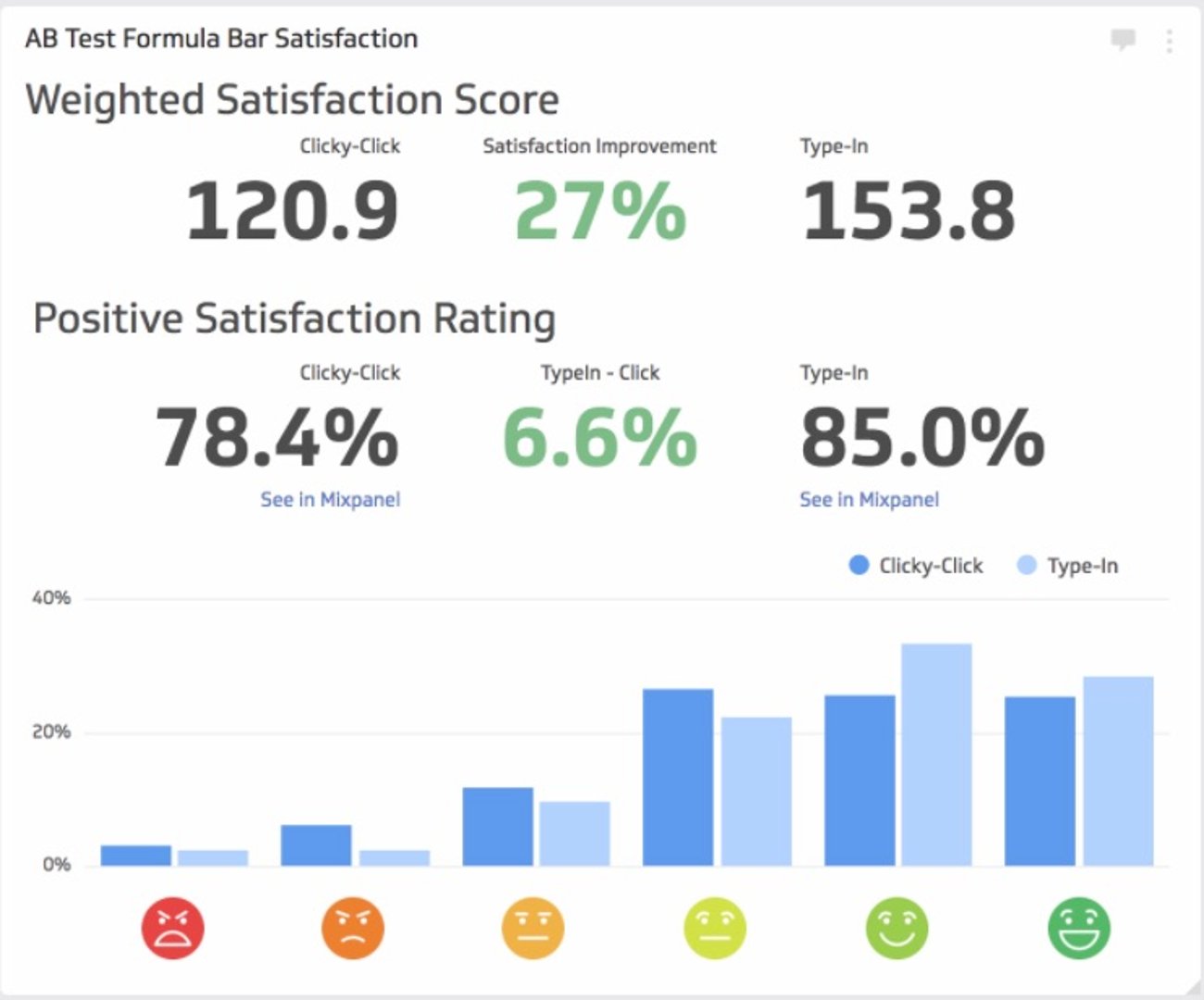
One of our insights was that users who are familiar with Google Sheets/Excel, or simply more skilled in our tool, enjoy manually typing their formulas. We built the type-in functionality for formulas and saw improved satisfaction compared to a button-only version of the interface.
Conclusion
UX research can help guide decisions, inform designs, derisk implementation and validate results. In addition, it’s really not hard to do and it doesn't need to be an expensive process. For those reasons, there is a lot of value in applying even a small amount of UX research at every stage of the development process.
Related Articles
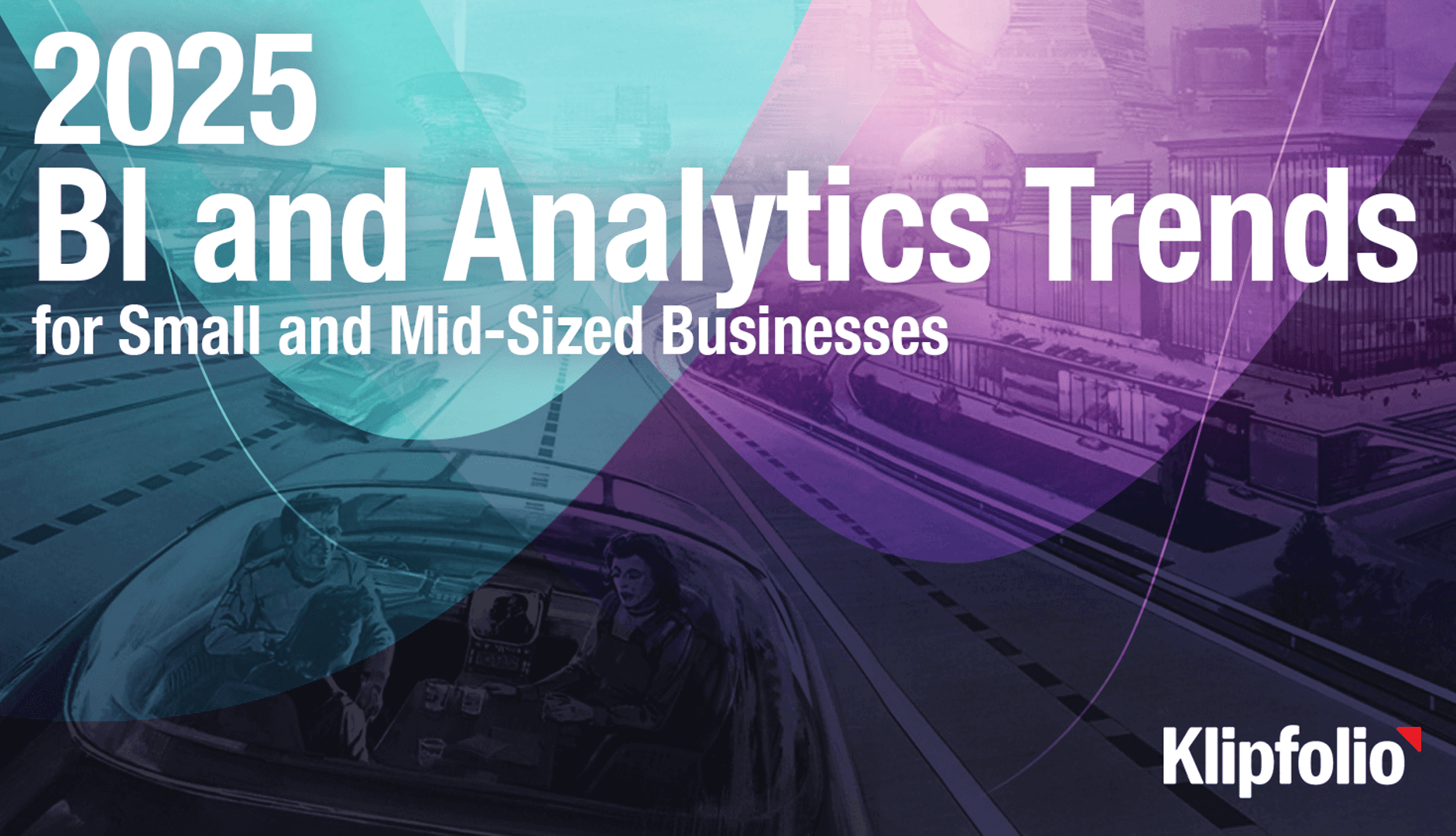
2025 BI and Analytics Trends for Small and Mid-Sized Businesses
By Allan Wille, Co-Founder — December 18th, 2024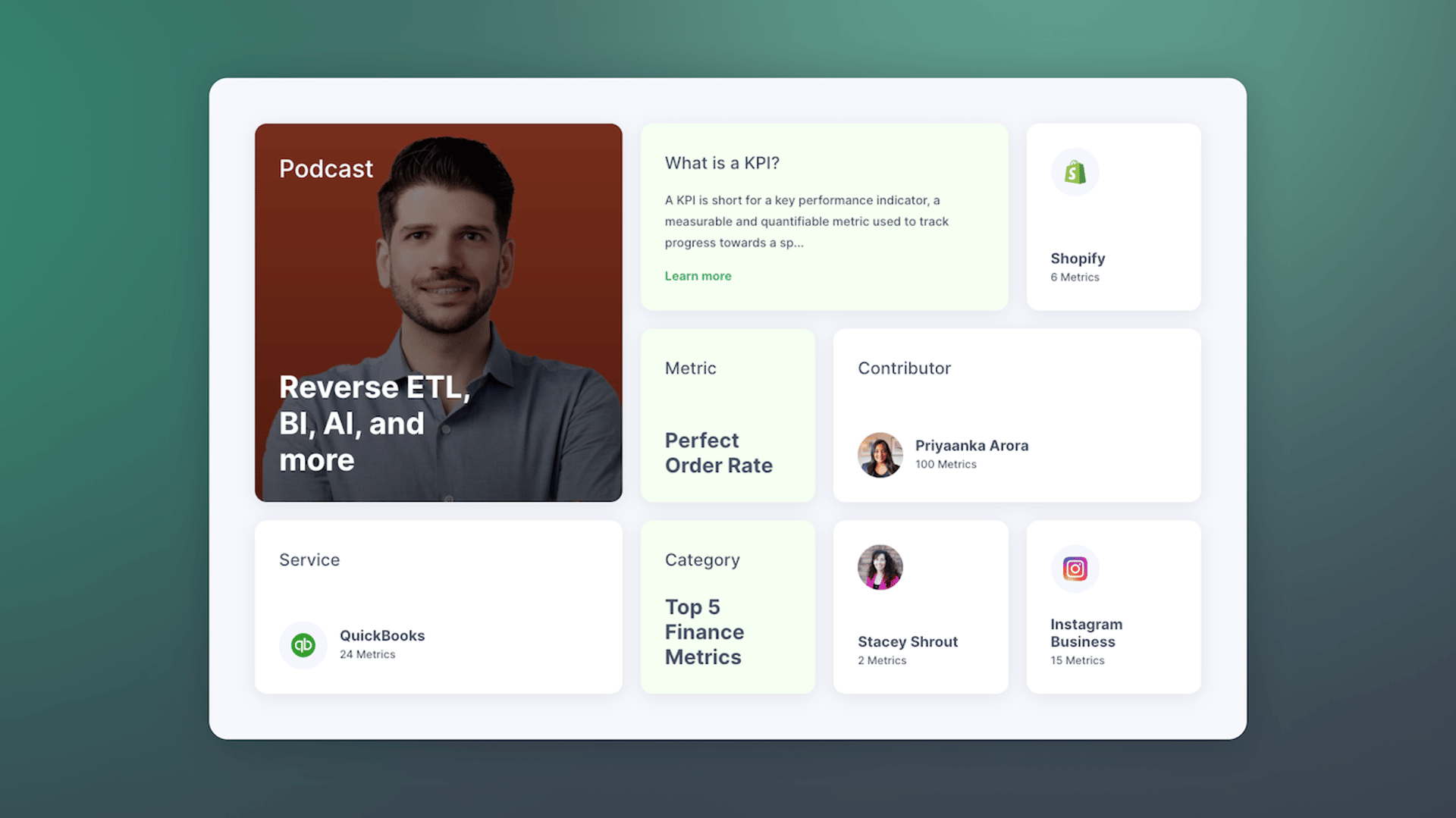
Promoting data literacy with metrichq.org and the power of AI
By Allan Wille, Co-Founder — October 12th, 2023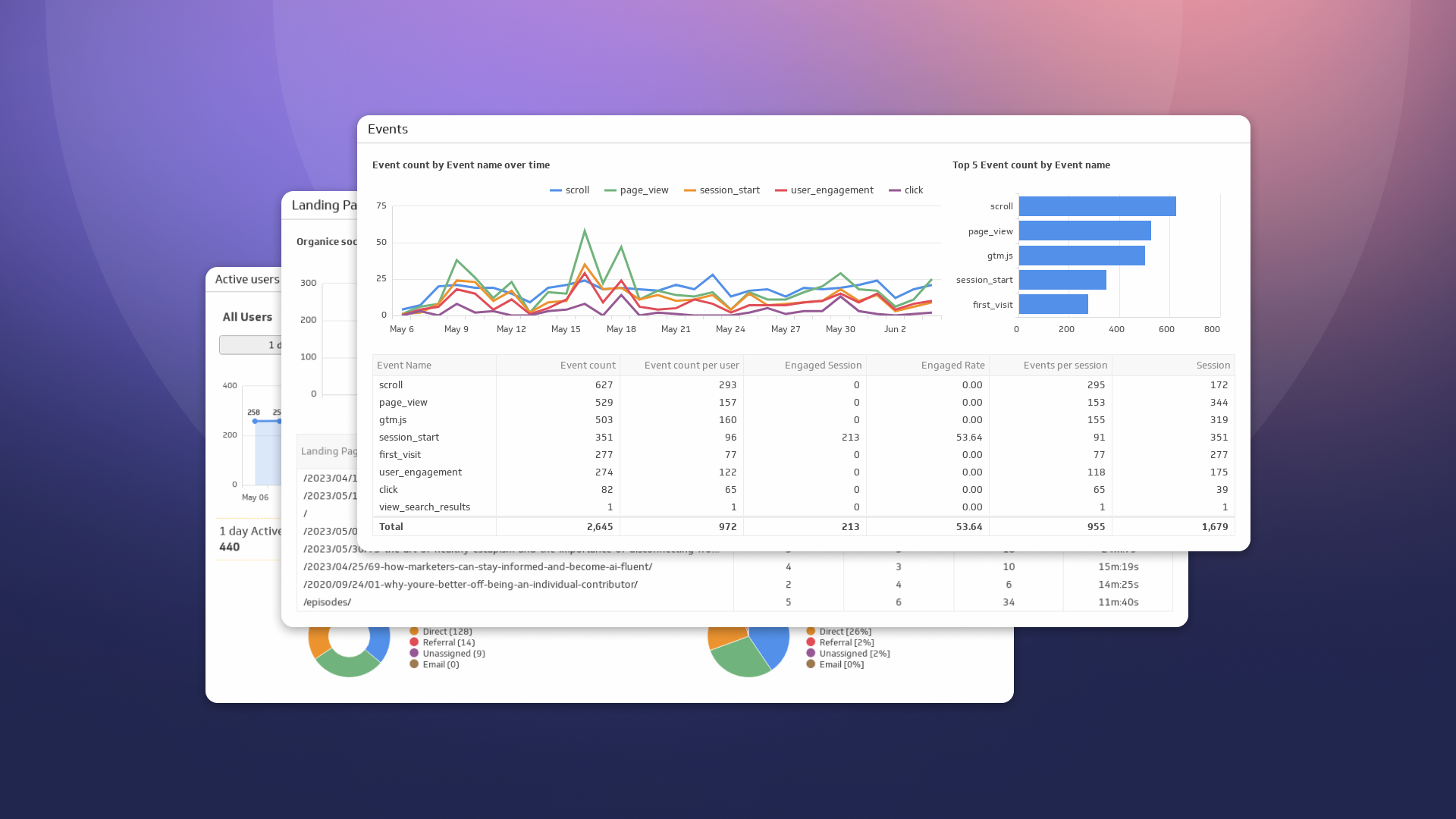
How to Build Google Analytics 4 Dashboards in Klips
By Jonathan Taylor — June 6th, 2023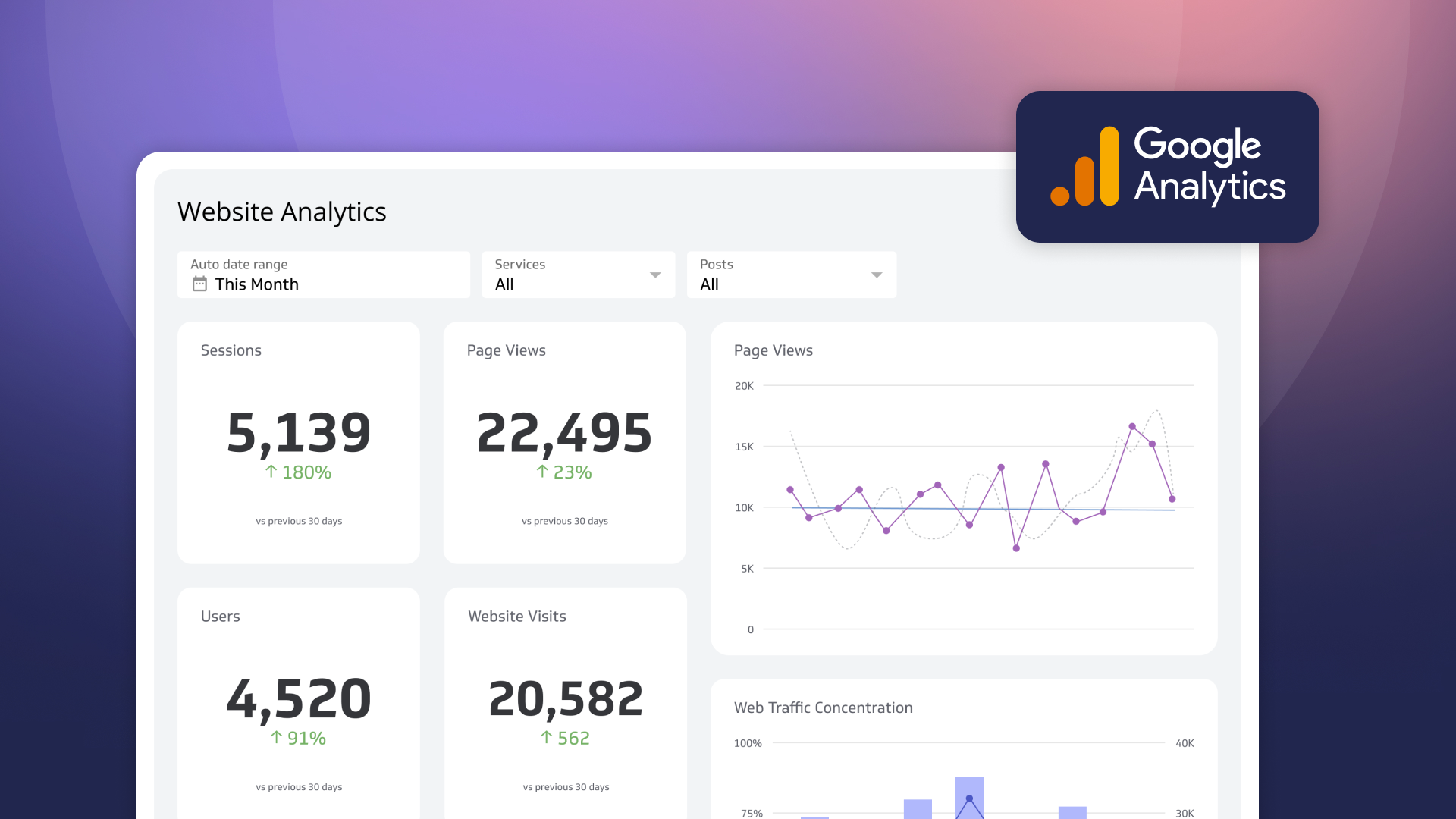